AREA IV: Networked teams
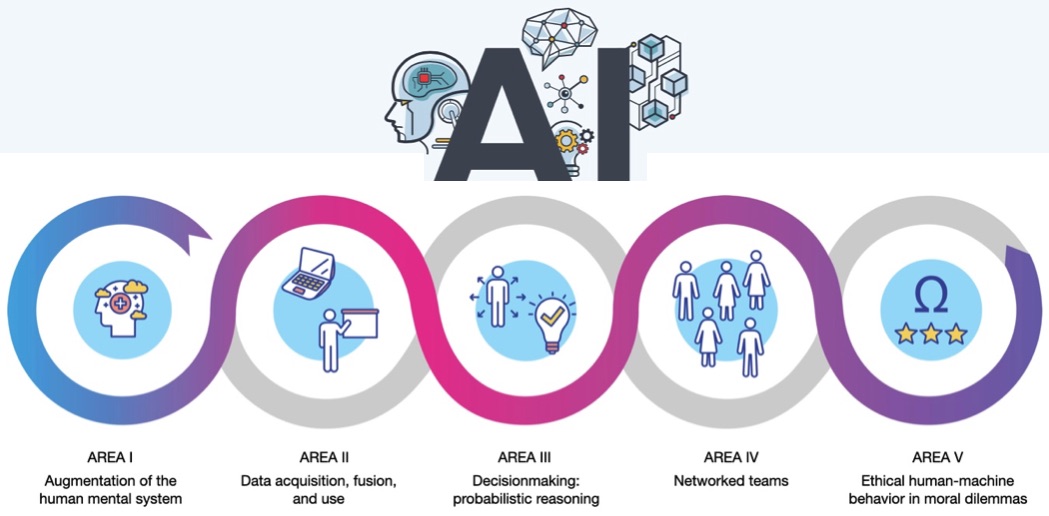
AREA IV Overview
General Principle: Design and engineering of smart machines for use in human-centered environments for supporting networked teams depends not only on advances from computer science and engineering but also from cognitive and systems science addressing the social cognition of networked teams. There is a difference in how people problem solve and make decisions independently and how problem-solving and decision-making takes place among networked team members (involving social cognition taking place in tightly or loosely-coupled organizational structures). On the surface, employing AI in support of networked teams would seem to offer advantages for distributive collecting and sharing large volumes of data and transcribing the data from one form to another in support of rapid processing needs by interdependent teams on a scale hardly imaginable just a few years ago. But well working networked teams accomplishing collective effort are especially difficult to quickly establish for high performance due to issues related to geographic separation distances among teams, differences in organizational cultures across teams, and the degree of trust present or absent among teams for others outside of the team.
Relevance for operational applications: Introducing smart machines for applications, in support of networked teams’ processing and contributions with decision-making chains, will require considerable care for not only the ‘mechanics’ associated with infrastructure/hardware integration for enabling distributive collection and sharing of large volumes of data but also careful consideration if networked teams do not trust input or output flowing from smart machines that are contributing critical assistance to a team’s processes and overall success. Generally, a team-of-teams approach towards timely, accurate orientation and interpretative work by networked teams, while necessary, has proven problematic in early stages of interdependent team effort, largely due to trust issues. Introducing smart machines for supporting or augmenting interdependent team networked processes will likely raise similar trust issues and may actually result in higher levels of mistrust among networked teams. Also, introducing smart machines for applications to support decision-making chains will require considerable care for not only addressing the ‘mechanics’ associated with infrastructure/hardware integration for enabling distributive collection and sharing of large volumes of data but also careful consideration of the impact on interdependent decision-making processes if networked teams do not trust the input or output flowing from contributions offered by smart machines. The likelihood of this occurring is even higher with networked teams offering little or no experience working together on interdependent effort across diverse organizational cultures and time zones.
Near term considerations: The employment of smart machines should not be done for supporting interdependent decision-making processes, associated with orientation and interpretation work by networked teams, without consideration of how humans depend on trust regarding another team’s input for their successful contribution on interdependent effort. Ample time is needed for gaining experiences with smart machines assisting with critical path processes that will be accepted and trusted by other teams and decision-makers. For now, dependence on the use of smart machines for supporting or augmenting interdependent effort among networked teams, contributing to critical path decision-making processes, ought to proceed with considerable caution when networked teams have little to no experience working on interdependent effort and trust among teams is in doubt. The time needed for networked teams to establish trust necessary for effective performance will increase pressure to increasingly use AI with humans out of the loop processes to gain perceived advantages associated with machine time. As highlighted in Area I, strategists and decision-makers will need to be aware and educated for developing their AI-metacognition to properly understand and account for risks when making decisions whether to keep or remove humans from AI-supported decision processes.