AREA II: Data acquisition, fusion, and use
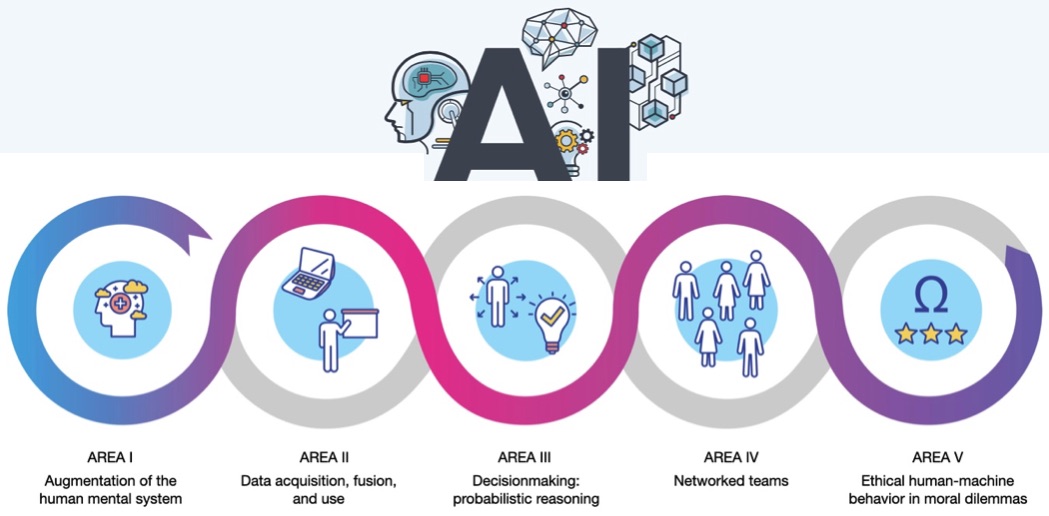
AREA II Overview
General Principle: Human use of data (datum) involves an observational point that characterizes a relationship of the value of a specific parameter for a particular object at a given point in time. Human interpretation of datum involves using formal and informal analysis to form knowledge. Formal analysis involves studies and models making use of mainly deductive reasoning. Informal analysis employs common sense, untested assumptions, and heuristics (rules of thumb) and employs mainly inductive reasoning. Human experiences and biases influence both formal and informal analysis resulting in knowledge. A computer created database can contain raw data from sensors but without any summarizing analysis. A knowledge base, on the other hand, is a collection of facts, heuristics, and models used for problem-solving and analysis of data. If the knowledge base provides sufficient structure, including semantic links among knowledge items (to include calculated neural net weights representing associations and frequencies) the computer itself may be able to apply that knowledge as an aid to problem-solving. When a computer (and those systems making use of AI) engages in these activities it is termed a ‘decision-support system’ (in cases involving the use of AI it is termed a ‘smart decision-support system’).
Relevance for operational applications: Selectivity (determining type and relevance of data desired for acquisition and usage) can benefit from rapid and improved accurate acquisition of data by machines but still requires humans-in-the-loop. Selectivity involves ongoing decision-making processes in combination by smart machines and humans that guide what is observed, data collected, structured and organized, and ultimately interpreted for relevance, decision, and action. How well this needs to be done in military applications is a matter of concern for roles expected by machines and humans since each will do it differently and interdependently. Data acquisition and usage will need to take place in consideration of what machines can do better and what humans can do better, and what can be done better when teamed together in roles leveraging the strengths of each.
Near term considerations: Current smart machine applications and tests involving the acquisition of data from sensor conduits in support of activities associated with human-centric observation and assistance with orientation of unfolding events have proven value. More is known, and applied in AI systems, about what smart machines can do better than humans with data acquisition and early AI processing conduits for initial orientation assistance to decision-makers. Less is known about iterative selectivity, cycles of data acquisition, and usage involving pairing of smart machines and humans (particularly under greater time/stressor pressures involving high risks).