AREA III: Decisionmaking: probabilistic reasoning
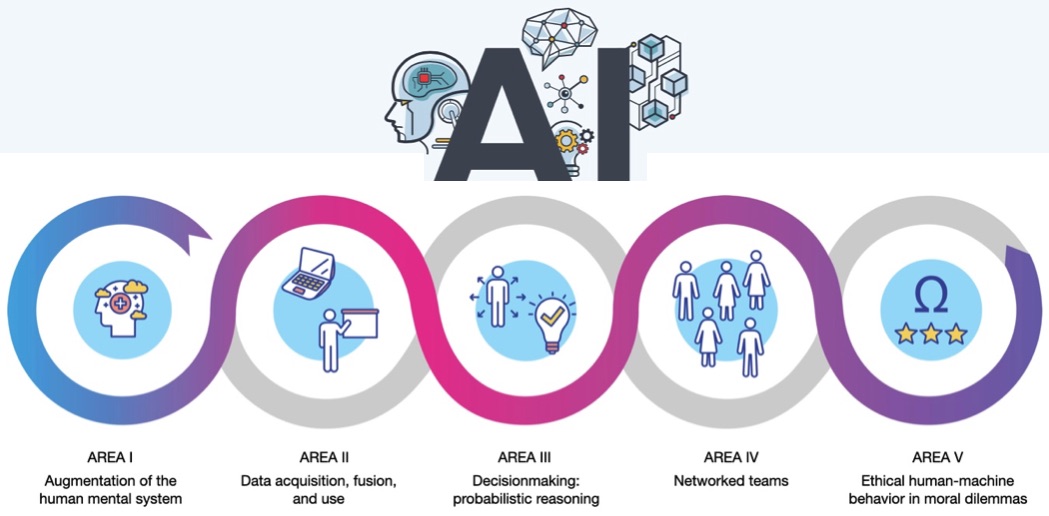
AREA III Overview
General Principle: Effective problem solving by humans employs combinations of formal and informal analysis at various levels along a continuum of encountered problem types; for example: whether a problem is commonly encountered, complicated (difficult to do but a ‘best’ solution can be discerned and acted upon because unknowns can become known), or complex (no definitive solution; unknowns resist becoming known resulting in decisions being made with incomplete knowledge of trade-offs between benefits and costs). Fundamentally, formal analysis done by humans, making use of deductive reasoning, is knowledge based employing hypothesis-directed processes of data collection, diagnosis, decision-making, action, review of results, and adaptation (referred to as System 2 or ‘slow thinking’). Informal analysis by humans contributes to problem solving by using inductive reasoning involved with sensemaking in light of past experiences for helping to initially frame problems for rapid response (referred to as System 1 or ‘fast thinking’).
Relevance for operational applications: Although humans can be taught to improve deductive and inductive reasoning skills we can expect decisionmakers to struggle with probabilistic reasoning associated with conditional events and deductive logic when encountering time pressures in an environment. Smart machines will do better than humans with probabilistic reasoning associated with conditional events when time, decisions, and action at the speed of machines would matter. Nonetheless, while smart machines can out-perform humans with probabilistic reasoning necessary for formal analysis we can expect human decision-makers will do a better job with informal analysis if given sufficient time. The expected use of AI-based decision-making, potentially employed with humans-out-of-the-loop, compounds the calculus for strategists for how best to address unfolding events occurring at the speed of smart machines. Unfortunately, results from research thus far conducted on human-machine teaming with AI in the face of escalating events have not been encouraging. Users of AI have struggled with keeping humans-in-the-loop when escalating events drive the need for decisions at the speed of smart machines. We don’t know enough yet about how to properly train future decisionmakers regarding AI-supported decision-making under stress.
Near term considerations: The speed of smart machines in stressful environments, wherein complex problems are very likely to be encountered with rapid unfolding of events presenting high risks, will require a combination of formal and informal analysis across decision-making chains. This will only be possible if human-machine teaming is employed with AI in applications with care in the overall design.